Good Failure, Bad Failure: A Framework for Evaluating Product Experimentation
This article explores the influence of product experiments and failures on product evolution. By categorizing them by learning and cost, we can assess their value.
Once upon a time, a stakeholder approached me with an idea to include a particular item in our product for the purpose of learning about our users' reactions. I suspected there was a hidden feature request behind their intentions. When I questioned the value of this exercise, asking "what would we learn from a potential failure?" The response was simply "we'd learn that the idea doesn't work". I couldn't help but ponder the worth of proceeding with this plan.
In effect, we can naively consider every feature we build as an experiment. Although they are not designed to fail, many of them fail. So failure deserves a lot of attention as a source of learning. And since failed experiments are inevitable, the wisest thing we can do is make them cheap and fast. In this blog post, I want to put a spotlight on product experiments and failures, and how they influence the evolution of product.
Anatomizing experiments and failures
From what I described in the previous paragraph, experiments can actually be categorised in two dimensions: the amount of learning we get from them and the price we pay for them.
Because failures are an overwhelmingly common and valid result of running experiments, they can also be categorized by the aforementioned dimensions. Presented here is a two-axis structure consisting of four spaces, each of which I will describe shortly.
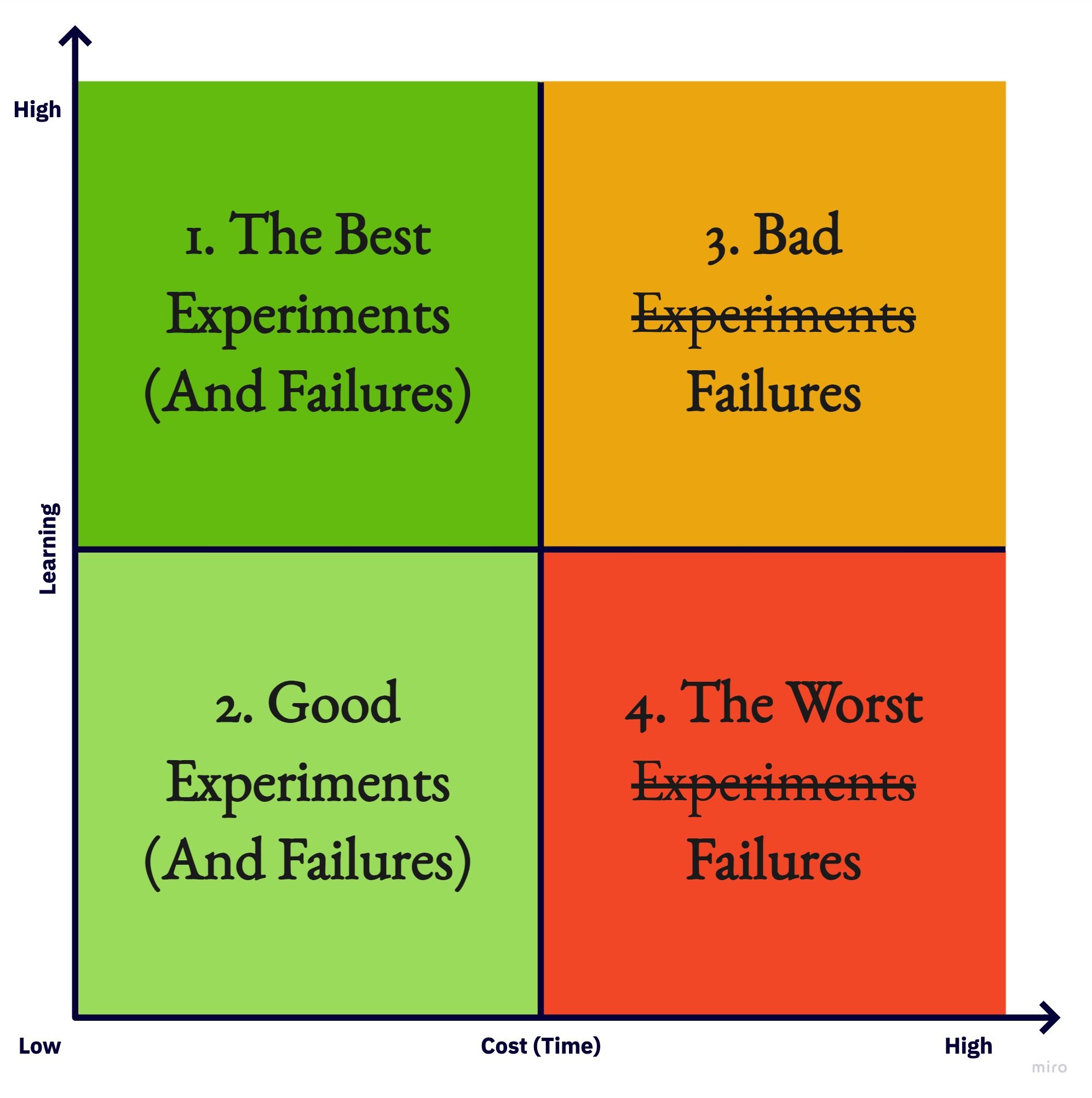
1. The best experiments and failures
The best experiments are very cheap and highly instructive. The value of what we learn from them is high because they can help us set strategic intents or shape product direction. They tell us more than just about optimization opportunities. They may contribute to the value proposition of our product.
Absolutely, a strategic decision cannot be made on the basis of a single test result. It takes time and requires mixing different sources of information to form a belief about the future of a product. Yet, the best experiments can profoundly alter how we view the current state of the product and how we set pieces next to each other to create the next product mutation.
These experiments are designed in response to the most consequential risks that may threaten the success of the product. At minimum, they help us discover the next critical questions we should answer to verify the problem we want to solve or evaluate the solution we want to deliver. Therefore, identifying the most important questions, assumptions or risks at every step of product development is mandatory for running the best experiments.
In other words, the true value of a piece of learning can only be estimated by analogy with other things we could have learned if we conducted another test. So when designing an experiment, we should think of the opportunity cost─the questions we could have asked, the things we could have learned or the experiment we could have run instead.
One thing that helps us in assessing the importance of a piece of learning is to contemplate the action we take after the result of an experiment is ready. This is one of the reasons that defining the next action is an integral part of designing an experiment.
2. Good experiments and failures
Good experiments or failures are inexpensive too, but if we watch the product direction from a high altitude, it is seldom shaped by these experiments or failures. They usually do not contribute to the product value proposition or provide groundbreaking insights, but they can inform tactical decisions or help us squeeze known opportunities to the hilt.
In other words, they are a very effective tool to capture the value that we have already created or discovered. By identifying bottlenecks in user flow, they may point to the next optimization opportunity, or through a simple change, they may enhance the conversion rate.
3. Bad experiments failures
Bad failures teach us valuable lessons about our product, customer or market. The reason we don’t like them is that they happen late or they are expensive. If you learn something valuable about your product, look for cheaper ways you could have used. This may help you spot a bad failure.
Being low cost is an innate characteristic of a product experiment. So in reality, we cannot blissfully call every feature an experiment. Even if we are lucky enough to deliver a successful feature without caring about what seriously threatens its success, we have failed to do our job by putting our team’s efforts and the company's resources at high risk.
If a few bad failures happen in succession, you may run out of resources to continue exploring other opportunities. Since these failures are about the building blocks of a business or an influential aspect of a product, they are more detrimental in the pre-product/market fit era.
4. The worst experiments failures
The worst failures also happen when teams do not take experimentation seriously. The reason they cannot elicit valuable information may be a lack of strategic thinking. When product teams want to learn significant things, they should have big questions to answer or challenging problems to solve. Often, big questions arise from strategic thinking about what will shape the direction of the product. This thinking includes prioritizing assumptions that should be validated first.
These failures may also be the result of investing time in incorrectly framed problems. When a problem is not framed properly, the product team takes great pain to find an answer to the wrong question. In such situations, even experiments that have a clear objective become pointless.
Feature factories are in imminent danger of such failures, but real product teams manage to avoid them.